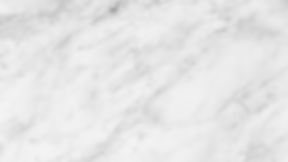
Bowen'
GIS Blog
Crime Analysis and GIS
Kumar, N. & Waylor, C. R. M. (2003). Proximity to Alcohol-Serving Establishments and Crime Probabilities in Savannah, Georgia: A Statistical and GIS Analysis. Southeastern Geographer, 43, 125-142.
​
Kumar and Waylor’s study (2003) presented an in-depth analysis of spatial distribution of crime in the city of Savannah, Georgia, and crime densities and probabilities in relation to their proximity to alcohol-serving establishments. The objective of their study was to use statistical and GIS analysis to determine if crime probabilities was correlated with proximity to alcohol-serving establishments. Kumar and Waylor hypothesized crime probability and density as inverse functions of distance from alcohol services, which expected that higher density and probability of crime would be closed to alcohol-serving establishments areas. The results of Kumar and Waylor’s analysis revealed that alcohol service vicinity had a strong impact on the spatial patterns of crime probability.
​
The study area was located in Savannah, Georgia because of the availability of geo-coded crime data. Geo-coding is a standard feature of police computer-aided dispatch (CAD) systems and makes data directly importable into any GIS databases. The city of Savannah had used this system since 1994 to track and record crime and related data. Therefore, Kumar and Waylor were able to obtain data on all crime incidents and their exact locations from the Police Department for the year of 2000. Moreover, the city had an exceptionally high crime rate of 4,615 per 100,000 for the US.
​
There were two main methods employed in this study which were random point generation and logistic regression, along with crime classification, crime density and crime probability determination. Kumar and Waylor grouped all crime into four categories: (1) Assault, extortion, blackmail, sex related, (2) Burglary, theft, robbery, larceny related, (3) Vehicle theft and related, and (4) Others. Crime density was calculated by the number of crime over geographic area of a given zone. Crime probability was calculated by number of crimes in a given zone to the total number of crimes and random non-crime locations in the same zone. This required randomly generated non-crime locations, so Kumar and Waylor used Grid Module of ArcGIS 8.1 to create a point layer of random points. Firstly, a mask of built-up area was assigned with random numbers, ranging in between 0 and 1. Then, the total number of pixels were counted in the built-up area. The authors used the cut-off value of random number (RNCOV) to select as many pixels as there were crimes, which there were 12,280 pixels approximately equal to the actual number of crime incidences. Lastly, the authors created a random point layer based on centroid coordinates of the 12,280 random pixels. Logistic regression was employed to assess crime probabilities in relation to the distance from the crime and random points to the closest alcohol serving establishments. The independent variable was distance from random locations and crime incidents to the closest alcohol services, and the dependent variable was crime and random location. Odds of crime occurrence were also calculated to indicate the probability of crime within 2,600 feet of distance.
​
The logistic regression result shows the probability of crime was an inverse function of distance from the alcohol serving establishments, in which regression coefficients are negative and statistically significant at 0.001 level for all four groups. Further, odds ratio for all crime was 0.33 which indicates that 67% decrease in the odds with one unit increase in the value of the natural logarithmic of distance. Distance from alcohol services explained one-quarter of the total variance, and three-quarters were unexplained, so the author concluded that alcohol-serving establishments can directly cause crime.
​
We would rate this paper 8 out of 10. The authors did an exemplary job in examining spatial dimensions of crime probability in relation to alcohol-serving establishments using GIS and logistic regression models. Consequently, the results of this study can make significant contributions to the Police Department to allocate resources efficiently. Further, the methods and models employed in this study could also be applicable to future crime analyses in relation to alcohol-serving establishments and other controlling variables in other places.